Chapter I. Pathogenesis
Rev Diabet Stud,
2012,
9(4):236-247 |
DOI 10.1900/RDS.2012.9.236 |
Metabolomics in the Studies of Islet Autoimmunity and Type 1 Diabetes
Matej Oresic
VTT Technical Research Centre of Finland, Tietotie 2, Espoo, FIN-02044 VTT, Finland
Manuscript submitted December 23, 2012; resubmitted January 21, 2013; accepted February 8, 2013.
Keywords: allostasis, autoimmunity, biomarker, lipid metabolism, lipidomics, metabolomics, systems biology
Abstract
The metabolome is sensitive to genetic and environmental factors contributing to complex diseases such as type 1 diabetes (T1D). Metabolomics is the study of biochemical and physiological processes involving metabolites. It is therefore one of the key platforms for the discovery and study of pathophysiological phenomena leading to T1D and the development of T1D-associated complications. Although the application of metabolomics in T1D research is still rare, metabolomic research has already advanced across the full spectrum, from disease progression to the development of diabetic complications. Metabolomic studies in T1D have contributed to an improved etiopathogenic understanding and demonstrated their potential in the clinic. For example, metabolomic data from recent T1D studies suggest that a specific metabolic profile, or metabotype, precedes islet autoimmunity and the development of overt T1D. These early metabolic changes are attributed to many biochemical pathways, thus suggesting a systemic change in metabolism which may be inborn. Based on this evidence, the role of the metabolome in the progression to T1D is therefore to facilitate specific biochemical processes associated with T1D, and to contribute to the development of a vulnerable state in which disease is more likely to be triggered. This may have important implications for the understanding of T1D pathophysiology and early disease detection and prevention.
Abbreviations: BCAA – branched chain amino acids; CE-MS – capillary electrophoresis coupled to mass spectrometry; C57BL/6 – mouse inbred strain C57 black 6; DIPP – Diabetes Prediction and Prevention (study); DNA – deoxyribonucleic acid; FADS1 – fatty acid desaturase 1 (gene); FTICR-MS – Fourier transform ion cyclotron resonance mass spectrometry; GC×GC-TOFMS – two-dimensional gas chromatography coupled to TOFMS; GC-MS – gas chromatography coupled to mass spectrometry; GF – germ-free; GWAS – genome-wide association studies; HDL-C - high-density lipoprotein cholesterol; HLA – human leukocyte antigen; IAA – insulin autoantibody; LC-MS – liquid chromatography mass spectrometry; mGWAS – GWAS combined with serum metabolomics; MS – mass spectrometry; MS/MS – tandem mass spectrometry; NMR – nucleic magnetic resonance; NOD - non-obese diabetic; PC – phosphatidylcholine; RNA – ribonucleic acid; SM – sphingomyelin; T1D – type 1 diabetes; TCA – tricarboxylic acid; TLR – toll-like receptor; TOFMS - time-of-flight mass spectrometry; XCMS – various forms (X) of chromatography mass spectrometry
1. Introduction
Metabolites are small molecules which are intermediates and products of metabolism. Glucose, amino acids, fatty acids, cholesterol, lactate, to give a few examples, all of them belong to the human 'metabolome'. The aim of metabolomics is to study the biochemical and physiological processes involving metabolites.
Studies of metabolites in the context of health and disease precede studies of genes. The very first known diagnostic application using metabolites can be tracked back 3000 years to China. Based on the observation that ants are attracted to sugar, the ants were utilized by ancient Chinese doctors to test urine in order to detect if the urine contained high levels of sugar [1]. In modern terminology, ants were applied as sensitive, albeit qualitative detectors of glucose, and hence to diagnose diabetes. Over the past 50 years, the core biochemical pathways such as the citric acid cycle (TCA cycle), glycolysis and gluconeogenesis, fatty acid and amino acid metabolism, and many others have become familiar to medical students attending basic biochemistry courses. In fact, much of the knowledge we have about metabolites today is still based on the pioneering biochemical and physiological studies over the period of 1950s-70s. Despite the technological limitations in this period, which enabled measurements of enzyme kinetics or metabolite concentrations only in an isolated manner, the 'system thinking' had already emerged [2]. Concepts such as 'metabolic control analysis' [3] were introduced, which viewed and modeled metabolism as a complex system of enzymatic reactions. For example, Henrik Kacser stated "But one thing is certain: to understand the whole you must look at the whole" [4]. The analytical strategy of studying a comprehensive 'profile' of metabolites in medicine was introduced by Linus Pauling and colleagues in the late 1960s, stating that "the thorough quantitative analysis of body fluids might permit differential diagnosis of many diseases in a more effective way than is possible at the present time" [5].
Over the past four decades, the emergence of molecular biology as a dominant tool of life science and biomedical research has contributed many new techniques for the study of genes and proteins, which inevitably changed the experimental paradigms in terms of how the biological systems are studied and how life scientists are educated. The ‘system thinking’ took a step back, giving way to a more reductionistic approach in life sciences where complex phenotypes were believed to be attributable to the function of specific genes and their products. Even today, this view dominates life science and biomedical research, although its limitations have been recognized [6]. Over the last decade, the ‘omics’ revolution has provided many new tools for comprehensive, quantitative, and sensitive measurements of molecular components of biological systems such as DNA, RNA, proteins, and metabolites as well as their interactions. However, the high-dimensional ‘omics’ data require a system biology approach, i.e., a shift in the focus from single components towards a collection of contributors in a network that make a specific complex phenotype [7]. This cannot be achieved using a reductionist experimental paradigm. In this regard, Joyner and Pedersen recently noted that the "…fundamentally narrow and reductionist perspective about the contribution of genes and genetic variants to disease is a key reason 'omics' has failed to deliver the anticipated breakthroughs" and they emphasized that the "critical utility of key concepts from physiology like homeostasis, regulated systems and redundancy as major intellectual tools" is necessary "to understand how whole animals adapt to the real world." [8].
In this review, recent advances in the study of the metabolome in health and disease are discussed, with specific focus on type 1 diabetes (T1D). It is argued that metabolomics is an analytical approach that is sensitive to multiple genetic and environmental factors which may together contribute to the development of the disease. As such an approach, it is an essential tool in the efforts to advance the etiopathogenic understanding and to develop novel approaches for T1D prediction, prevention, and treatment.
2. Analytical strategies for matebolomics
The human metabolome comprises 1000s of small molecules of high chemical diversity across a wide dynamic range of concentrations. The latest edition of the Human Metabolome Database, version 3.0, contains 40,271 metabolite entries [9]. It is thus not surprising that no single analytical approach can cover all metabolites within a single analysis. For a comprehensive overview of metabolomic methods in life sciences and medicine, the reader is referred to an excellent recent review by T. Hyötyläinen [10]. A summary of typical metabolomic strategies is provided below.
A typical metabolomics pipeline is illustrated in Figure 1. The initial steps are sampling and sample handling, which are critical for the success of the subsequent analysis. No matter which analytical methods are applied, reliable results cannot be obtained if the samples have not been handled properly. The critical steps, non-exclusively, include sample drawing and transport, sample storage, and the amount of freeze-thaw cycles. While some metabolites such as bile acids tend to be rather stable in different sample handling conditions, others including eicosanoids are extremely sensitive to any of these steps, requiring special sampling and sample preparation protocols. Careful consideration and proper quality control of the analytical steps are essential to minimize analytical variation, and thus to detect the relevant biological variation.
 |
 |
Figure 1. Schematic illustration of a typical metabolomic pipeline. For each pipeline stage (dark boxes), specific key factors to consider and procedures involved are listed as examples (light boxes). |
|
For the analytical determination, the hyphenated approaches based on liquid chromatography mass spectrometry (LC-MS), gas chromatography coupled to mass spectrometry (GC-MS), and to a lesser extent capillary electrophoresis coupled to mass spectrometry (CE-MS) have commonly been applied for sensitive metabolomic analyses of tissues and biofluids. Techniques based on direct infusion, such as microfluidic-based nanoelectrospray ionization [11], have been used in the so-called 'shotgun lipidomics' approach [12, 13]. This approach enables the determination of hundreds of molecular lipids without some of the inherent problems of LC-MS such as sample carry-over. However, this advantage is achievable only at the cost of lower sensitivity and greater challenges with quantification. Another metabolomic technology is proton nucleic magnetic resonance (1H NMR); it is chemically non-selective and requires only minimal sample preparation. The cross-laboratory validation of the NMR method is relatively uncritical; MS-based approaches are more challenging in this respect. However, NMR is about three orders of magnitude less sensitive than MS-based methods. It is thus capable of detecting only the most abundant metabolites. The facilitation of reproducibility has contributed to the emergence of NMR as a popular approach for epidemiological studies [14, 15]. While the required sample amount in NMR is rather high (typically about 300μl of serum, compared to 10-30μl in MS-based analysis), and the interpretation of NMR signals in the context of molecular and clinically relevant markers is rather challenging and still unresolved, developments are under way which may help to characterize the NMR-associated traits with the help of MS [16].
Recent developments in MS-based approaches provide improvements both in the comprehensive coverage of metabolites and the ability to quantify them. The emerging multi-dimensional techniques such as two-dimensional gas chromatography coupled to time-of-flight mass spectrometry (GC×GC-TOFMS) have a high-peak capacity. Thus, they enable sensitive detection of 1000s of different metabolites within a single sample run, and quantification of selected metabolites within the same run [17], requiring only about 20 μl of serum sample for the analysis.
Metabolomic strategies have commonly been divided into 'targeted' and 'non-targeted' approaches. The targeted methods focus on accurate (usually quantitative) determination of a pre-determined set of metabolites, while the non-targeted methods are optimized for maximal coverage of the metabolome, although at the cost of lower sensitivity and ability to achieve reliable quantification. The boundary between the two approaches is disappearing because recent technological developments enable a combination of both targeted and non-targeted strategies in a single analysis. For example, the abovementioned GC×GC-TOFMS can be set up to absolutely quantify several tens of metabolites, while semi-quantitatively determining 1000s of metabolites [17]. If any of the semi-quantified metabolites turns out to be biologically important based on the results of the analysis, it can later be included in the panel of quantified metabolites. Similarly, the LC-MS-based lipidomic approaches, used for the analysis of molecular lipids, can cover a pre-determined set of lipids, while also including the ‘non-identified’ peaks which can be identified in follow-up analytical experiments if considered of interest [18].
The identities of metabolites can be determined by using tandem mass spectrometry (MS/MS) and to a limited extent also by accurate mass measurements using innovative algorithms for molecular formula calculations [19]. The latter is facilitated by the availability of new high-resolution and accurate mass instruments such as Fourier transform ion cyclotron resonance mass spectrometry (FTICR-MS) and the Orbitrap. However, the LC-based methods cannot be easily reproduced across different laboratories, thus the standardized library-based identification of metabolites is challenging. In contrast, large libraries of spectra have been collected over the past decades for GC-MS based methods.
Data processing is a critical step in metabolomic analysis which converts the signal from the analytical instrument into biologically meaningful information [20]. Specialized software packages exist, including the popular open source platforms MZmine [21] and XCMS (acronym for various forms (X) of chromatography mass spectrometry) [22]; both of which enable the fine tuning of the data processing pipeline to the needs of the specific analytical approach. The advantage of open source applications for data processing is that their mode of operation is accessible and they are not 'black boxes'. This allows improved quality control and better understanding of how the instrumental signal is transformed into biological information. The overall strategy of subsequent data analysis is similar to those used in other 'omics' approaches, and requires similar statistical considerations due to the typical high dimensionality of the data [23].
The interpretation of the metabolomic data in the physiological context remains a challenge, in particular those data obtained from serum (or plasma). The concentration of a specific metabolite in the blood is typically a balance of several organ-specific incoming and outgoing fluxes. Based on a single metabolite measurement, it is nearly impossible to determine which specific physiological phenomenon corresponds to an abnormal change of the specific metabolite. In addition to individual metabolites, it is thus advantageous to study the patterns of metabolite changes, e.g., those corresponding to similar functional groups or biochemical pathways [24]. In this context, lipids are particularly challenging because the major molecular lipids commonly measured by lipidomics approaches, such as triglycerides and phospholipids, are major constituents of lipoprotein particles (when measured in blood), or of biological membranes and lipid droplets (when measured in cells and tissues). Therefore, biochemical pathway-level descriptions are not sufficient for the proper interpretation of lipidomic data. Instead, the data should be interpreted in the context of the biophysical systems they belong to, such as lipoproteins or membranes [25]. To facilitate the interpretation of lipidomics data in the biophysical context molecular simulation approaches have been introduced recently [26, 27]. However, it is fair to say that the methods for interpreting the metabolome, which include the lipidome at the system level, are still in their infancy.
3. Factors influencing the metabolome
It is becoming clear that, despite the undeniably strong genetic component of many complex diseases including T1D, the current approaches used to study the genetic association with disease traits can explain only a fraction of the known disease heritability [28]. Such a discrepancy is not a surprise to system biologists, who view the development of a complex disease as a dynamic process, involving gene-environment and molecular interactions across many physiological levels. In this context, the measurement of those traits that are not encoded by the host genome directly, commonly referred to as intermediate phenotypes [29], is particularly important.
Genome-wide association studies (GWAS) have been commonly applied to link specific genetic variants with the disease endpoints. However, the strength of the so-found associations is generally weak, even if statistically highly significant. Furthermore, little information about the underlying disease mechanisms can be obtained from direct associations between the genetic variants and disease phenotypes. To overcome these shortcomings, GWAS have recently been combined with serum metabolomics. These approaches, commonly referred to as mGWAS (recently reviewed in [30]), have already demonstrated the potential of using concentrations of circulating metabolites as intermediate phenotypes [31-33]. For example, in the first application of this kind, Gieger et al. have shown that polymorphism rs174548 in the FADS1 gene encoding fatty acid delta-5 desaturase is associated with the ratios of phospholipids containing long-chain polyunsaturated fatty acids [31]. The ratios likely correspond to the ratio of fatty acids C20:4 versus C20:3, with the explained variance larger than 30%. The association of FADS1 with specific phospholipids may provide mechanistic insight into the diseases known to be associated with the FADS1 locus, e.g., Crohn’s disease [34] and lipid disorders [35], and suggests that the availability of polyunsaturated fatty acids and their incorporation into the membrane phospholipids play a role in these disorders.
It is now widely acknowledged that our host genome comprises only a small fraction of the total gene pool in the human body, which also includes genomes of the complex microbial ecosystem located in the distal gut [36]. This microbial ecosystem has coevolved with the host and developed traits that would not have developed on their own, including vitamin production, xenobiotic metabolism, fermentation of complex polysaccharides, and education of the immune system [36]. However, not all of these functions are beneficial to us as altered gut microbiota characterizes many diseases [37]. Changes in the microbiota have been shown to affect the metabolome of the host, suggesting that it plays an important role in host physiology [38]. Germ-free (GF) mice have a drastically altered serum metabolome and host lipid metabolism [39, 40]. However, it is generally difficult to discern whether an altered microbiota, as observed in many diseases, is a cause or consequence of the changes in host metabolism and disease progression, and how it contributes to the disease. Given the complexity of the microbial ecosystems and its interactions with the host, it is thus even more essential than in GWAS that studies seeking correlations of gut microbial variation with disease endpoints are accompanied by integrative approaches, including measurements of intermediate phenotypes, which help to provide mechanistic insights into the observed associations [41].
Studies in mice suggest a direct involvement of the gut microbiota in T1D development. The adapter molecule Myd88 that transmits signals from toll-like receptors (TLRs), which function as pattern recognition receptors, is an important regulator of gut microbial ecology. Non-obese diabetic (NOD) mice deficient in Myd88 are resistant against T1D development via a mechanism that is mediated by an altered gut microbiota [42]. Investigations in NOD mice have also shown that lack of gut microbiota promotes an imbalance between Th1, Th17, and Treg differentiation in the intestine [43] and affects sex hormone levels [44], together promoting a more diabetogenic environment. Progression to autoimmune diabetes, in conventionally raised NOD mice, has been associated with reduced diversity of the butyrate-producing bacteria of the Clostridium leptum group [45]. Interestingly, diminished diversity of the anti-inflammatory commensal bacterium Faecalibacterium prausnitzii from the C. leptum group is also a determinant of Crohn's disease [46]. Furthermore, a recent study revealed that microbes from the C. leptum group induce regulatory T cells in the colonic mucosa [47], thus implicating this microbial group in the regulation of immune homeostasis. Recent data also suggest that children who later progress to T1D have an altered microbiota with a deficit in butyrate-producing bacteria [48, 49].
In addition to host genome and gut microbiota, the metabolome is also sensitive to other factors related to life-style and life-course, including development [50], age [51], immune system status [52, 53], and diet [54]. This makes metabolomics a key platform in medical system biology, particularly as a sensitive phenotyping platform that detects and integrates the genetic and environmental factors which together affect the progression to complex diseases, including T1D [55] (Figure 2).
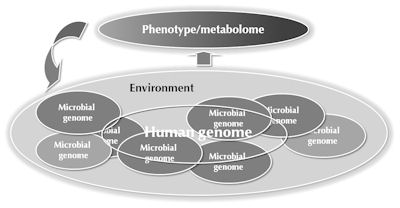 |
 |
Figure 2. Factors influencing the metabolome. The metabolome is sensitive to genetic and environmental factors which may together contribute to the disease. Metabolomics is thus a powerful phenotyping platform in biomedical studies. |
|
4. Metabolomic approaches to study type 1 diabetes
Given the sensitivity of the metabolome to several pathogenically relevant factors, it is not surprising that metabolomics has been increasingly applied in biomedical research. For example, metabolomic approaches are used to detect novel biomarker candidates for:
- Prostate cancer [56]
- Progression to type 2 diabetes [57-59]
- Alzheimer's disease [60]
Also metabolomics are applied to:
- Identify novel targets of cancer [61]
- Monitor stem cells in regenerative medicine [62]
- Determine responses to chemotherapy [63].
In contrast to the study of type 2 diabetes, where an increasing number of metabolomic investigations is being performed [64], metabolomics has been a rather minor component in T1D research. This may be due to the fact that T1D has been widely considered as an immune-mediated disorder with a major genetic component, and the role of metabolism in disease progression besides the diagnosis of T1D has not been appreciated in general. Recent and ongoing metabolomic studies and the increasing recognition of the role of gut microbiota in T1D progression are likely to change this outlook.
Albeit scarce, metabolomic studies in T1D have already covered the full spectrum of progression to the disease and the development of diabetic complications. Comprehensive metabolomic approaches (LC-MS for detection of molecular lipids and GC×GC-TOFMS for detection of polar metabolites) were applied to a longitudinal series of samples taken between birth and onset of overt T1D from children enrolled in the Finnish Type 1 Diabetes Prediction and Prevention (DIPP) study [52]. Compared to the controls matched for gender, HLA risk, period and city of birth, children that later progressed to T1D had decreased phosphatidylcholines (PCs) at birth (cord blood) and persistently diminished ether phospholipids during the follow-up. Decreased PCs in cord blood may be pathogenically important because choline, which is mainly incorporated in PCs in a non-free form, is in particularly high demand during pregnancy as a substrate for building cellular membranes due to rapid fetal tissue expansion and increased production of lipoproteins [65]. Furthermore, choline is a major provider of methyl groups needed for DNA methylation, and is therefore essential for developmental processes, including genomic imprinting and the maintenance of genome stability [65, 66].
In the same DIPP metabolomic study, the appearance of first islet autoantibodies was preceded by increased levels of proinflammatory lysophosphatidylcholine (lysoPC), glutamate, and branched chain amino acids (BCAAs), and decreased levels of several TCA cycle metabolites. Interestingly, the appearance of autoimmunity normalized the metabolic profiles to the levels found on average in control children [52]. While these findings need to be validated further, they do point to the potential application of metabolomics in clinical practice. Inexpensive diagnostic assays could be developed to measure the selected key metabolites, which could be applied together with genetic and islet autoantibody screening to identify children at increased risk of T1D.
In the German BABYDIAB study, metabolic profiles were studied in relation to the age of onset of islet autoimmunity [53]. The key finding of the study was that significant differences in metabolite profiles were observed relative to age and islet autoantibody status. For example, the children who developed autoantibodies by age 2 years had a twofold lower concentration of methionine compared with those who developed autoantibodies in late childhood or who remained autoantibody-negative. This is in agreement with the metabolomic results of the DIPP study [52], because in the DIPP study most of the children progressed to islet autoimmunity during their first years of life, and because progression to T1D and not to islet autoimmunity per se was the primary endpoint. One of the open key questions from these studies is therefore whether the observed early metabolic signature which precedes autoimmunity is (a) specific to those children who later progress to T1D or (b) more broadly found in children who later progress to one or more islet autoantibodies.
A study in NOD mice using a prospective design similar to that of the human DIPP study showed that female mice which later progress to autoimmune diabetes share a similar metabolic profile with children who later progress to T1D [45]. Based on the measurement of insulin autoantibody (IAA) and serum lysoPC, a surrogate marker was developed, which stratified the mice according to their risk of diabetes. In a follow-up study, mice identified to be at high risk of autoimmune diabetes had more advanced insulitis and higher concentrations of several amino acids, including the insulinotropic BCAAs and glutamate in the islets [45] (Figure 3). While still normoglycemic, these high-risk prediabetic mice also had increased glucose-stimulated insulin secretion and, as already stated, a diminished diversity of the C. leptum microbial group in the gut. Together, these findings indicate that autoimmune diabetes is preceded by a state of increased metabolic demands on the islets, resulting in elevated insulin secretion. They also support the hypothesis that the metabolic profile found in the DIPP prediabetic children is specifically associated with progression to T1D.
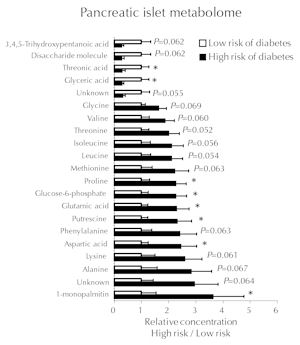 |
 |
Figure 3. Metabolic profiles. Selected metabolic profiles in isolated islets from female NOD mice at low versus high risk of developing autoimmune diabetes. A total of 128 metabolites were measured. Reproduced with permission from Sysi-aho et al. [45]. |
|
Metabolomic approaches were also applied to compare prospective blood samples from female NOD mice with those from the C57BL/6 (wild type) mice [67]. Partly resembling the results from the DIPP study [52], NOD mice had a distinct metabolic profile which included increased glutamate and decreased TCA cycle metabolites. The subsequent in silico analysis indicated that the genes responsible for this reside within previously defined Idd regions [67].
Together, the initial findings from the early stages of T1D development in humans and mice suggest an important role of the metabolome in early disease pathogenesis. They also provide a compelling case for further investigations using a systematic approach in the prospective clinical cohort studies, which combines metabolomics with (epi)genetic profiling (e.g., the mGWAS approach), gut microbial characterization, and more detailed characterization of immunologic processes including immune reactivity.
Overt T1D often leads to severe medical complications, the so-called chronic micro- and macrovascular complications of diabetes. It would be clinically important to identify the patients at risk of developing such complications. Early markers would provide new tools for diagnosis and improvement of patient monitoring, novel insights into the etiopathogenesis of diabetic complications, and thus novel targets for prevention or treatment. NMR-based metabolomics has been applied to study T1D patients at risk of developing diabetic complications in the Finnish FinnDiane study [68-71]. One of the key findings of this line of research was that progression to diabetic complications such as nephropathy is complex and involves interactions of multiple metabolic and clinical factors, including insulin resistance and the metabolic syndrome [68, 71]. This emphasized the importance of adopting a multi-modal approach to predict and diagnose diabetic complications in the clinical setting. One of the unexpected findings from the FinnDiane study was that high-density lipoprotein cholesterol (HDL-C) is one of the predictors for all-cause mortalities in adults with T1D [72]. This paradoxical role of HDL in T1D patients is unclear, further supporting the emerging idea that serum HDL-C levels alone may not be sufficient for the atheroprotective role of HDL particles. Of potential relevance, sphingomyelin (SM) was found to be associated with diabetic kidney disease in the FinnDiane study [69]. In a general population study, SM was found elevated in HDL fractions of healthy subjects with high HDL-C [27]. Together, these findings suggest that the association of HDL-C with increased mortality in T1D patients may in part be mediated by changes in lipid composition, and consequently the function of HDL particles. This reiterates the importance of comprehensive lipidomics approaches for a more detailed understanding of HDL particles in the development of T1D and its complications.
5. The metabolome as a factor in the progression to type 1 diabetes
To discuss the potential etiopathogenic role of the metabolome in T1D, this section returns to the metabolomic findings from the DIPP study [52] and from NOD mice [45]. In addition to the aforementioned question regarding the disease specificity, another open question is what are the pathogenic role and related mechanisms behind the observed metabolic changes in early progression to T1D. Put differently, is the metabolome a bystander of the disease process and a correlate of other still unknown pathogenic phenomena, or does it play an active role in the disease process? While these two possibilities are nonexclusive, an argument is presented here for the latter scenario.
Although still limited, current evidence from humans and mice suggests that 'metabolic stress' precede the appearance of the first islet autoantibodies, i.e., a metabolic profile (or 'metabotype') that includes elevated insulinotropic BCAAs and glutamate, and diminished phospholipids and energy (TCA cycle) metabolites. This metabotype cannot be explained by diet alone, even not in human studies [55]. Given the degree of changes, across metabolites representing many different functional classes and pathways, it is unlikely that the metabotype is attributed to a specific biochemical pathway. Based on the persistence of the metabolic changes observed in the DIPP study [52], a more likely explanation is that this metabotype is inborn, i.e., inherited or acquired prenatally or during the first months of life. In indirect support of this view, lipid-bound choline, a major donor of methyl groups during pregnancy [65], was found decreased in cord serum of newborns that later progressed to T1D. A potential contributing role of gut microbiota, including that of the mother, to this metabotype cannot be ruled out. It has been noted previously that the observed cord serum metabolic profiles in T1D progressors (as compared to controls) are similar to the metabolic profiles of germ-free mice (as compared to conventionally raised mice) [39].
The possible pathways leading to the observed prediabetic metabotype may in fact be manifold, but once acquired, this metabolic profile contributes to a state of increased metabolic demands on the islets to maintain their normal metabolic function. These physiological changes, aiming to maintain the normal healthy state, can be considered as an adaptation, or allostatic change (Figure 4). Typically, such allostatic adaptations, which may include autoimmune responses [73, 74], occur to induce short-term corrective changes to regulatory systems. However, when activated for long periods of time, the maintenance of metabolic homeostasis might actually be achieved at the expense of a metabolic cost, or 'collateral damage', defined by McEwen as allostatic load [75]. The allostatic load derived from the allostatic adaptations, in combination with a specific genetic make-up (e.g., high HLA-associated risk of T1D), and in the context of specific developmental stages might define different degrees of ‘vulnerability to disease’, as illustrated by the lowered threshold between the healthy state and T1D in Figure 4. Once the state of increased 'disease vulnerability' is acquired, the paths to T1D may again be manifold, initiated by one or many of the environmental triggers of T1D [76]. In this view, the metabolome can be regarded as a key mediator between disease initiation and both the genetic risk and related immune dysfunction.
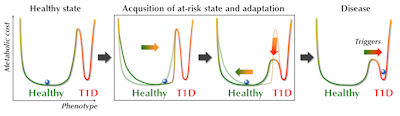 |
 |
Figure 4. Hypothetical progression towards T1D in the context of the metabolome. The acquisition of an at-risk state is followed by a stage of adaptation aimed at maintaining the 'normal healthy state'. This adaptation may occur at a cost of increased vulnerability to disease, i.e., the threshold for specific triggers to initiate the disease process is lowered. |
|
6. Conclusions and future perspectives
In summary, the metabolome is sensitive to genetic and environmental factors and thereby contributes to complex diseases such as T1D. This makes metabolomics one of the key platforms for the discovery and study of pathophysiological phenomena leading to T1D and the development of T1D-associated complications. Currently available metabolomic data from T1D studies suggest that a specific metabotype precedes islet autoimmunity and the development of overt T1D. Based on this evidence, the role of the metabolome in progression to T1D is therefore a key contributor to the acquisition of a vulnerable state, which is more likely triggered towards disease initiation, than a specific biochemical process associated with T1D. While clearly much more research and data are needed to prove this hypothesis, it may have important implications for early disease detection and prevention. For example, the question may be asked why some individuals at increased risk of T1D, i.e., those with increased HLA risk and 1-2 detected islet autoantibodies, do not develop T1D. Do they have a metabotype different from those who later progress to disease? If so, can T1D-associated metabotypes be modulated, e.g., by altered diet?
Given the dynamic involvement of many physiological systems in progression to T1D, future investigations of T1D pathogenesis and etiology will have to adopt an integrative system biology approach. This will require intensive data acquisition and the construction, refinement, and validation of mathematical models that include many contributing factors across multiple spatial and temporal scales [74, 77]. Metabolomics is likely to provide some of the key data needed to understand the complex dynamics leading to T1D.
Disclosure: The author reports no conflict of interests.
Acknowledgments:
This work was supported by the Academy of Finland Centre of Excellence in Molecular Systems Immunology and Physiology Research (SyMMyS; Decision No. 250114). I also thank Mikael Knip and Olli Simell as well as the staff of the VTT Quantitative Biology and Bioinformatics group for excellent discussions and contributions to the metabolomic studies in type 1 diabetes.
References
- Greef J, Smilde AK. Symbiosis of chemometrics and metabolomics: past, present, and future. J Chemometr 2005. 19:376-386. [DOD] [CrossRef]
- von Bertalanffy L. General systems theory. 1969, New York, George Braziller. [DOD]
- Kacser H, Burns JA. The control of flux. Symp Soc Exp Biol 1973. 27:65-104. [DOD]
- Kacser H. On parts and wholes in metabolism. In: Welch GR, Clegg JS (eds). The organization of cell metabolism.1986, New York, Plenum Press. [DOD]
- Pauling L, Robinson AB, Teranishi R, Cary P. Quantitative analysis of urine vapor and breath by gas-liquid partition chromatography. Proc Nat Acad Sci U S A 1971. 68:2374-2376. [DOD] [CrossRef]
- Lazebnik Y. Can a biologist fix a radio? Or, what I learned while studying apoptosis. Cancer Cell 2002. 2:179-182. [DOD] [CrossRef]
- Ideker T, Galitski T, Hood L. A new approach to decoding life: systems biology. Annu Rev Genomics Hum Genet 2001. 2:343-372. [DOD] [CrossRef]
- Joyner MJ, Pedersen BK. Ten questions about systems biology. J Physiol 2011. 589:1017-1030. [DOD] [CrossRef]
- Wishart DS, Jewison T, Guo AC, Wilson M, Knox C, Liu Y, Djoumbou Y, Mandal R, Aziat F, Dong E, et al. HMDB 3.0 - The Human Metabolome Database in 2013. Nucleic Acids Res 2013. 41:D801-D807. [DOD] [CrossRef]
- Hyotylainen T. Novel methodologies in metabolic profiling with a focus on molecular diagnostic applications. Expert Rev Mol Diagn 2012. 12:527-538. [DOD] [CrossRef]
- Stahlman M, Ejsing CS, Tarasov K, Perman J, Boren J, Ekroos K. High-throughput shotgun lipidomics by quadrupole time-of-flight mass spectrometry. J Chromatogr B 2009. 877(26):2664-2672. [DOD] [CrossRef]
- Han X, Gross RW. Shotgun lipidomics: electrospray ionization mass spectrometric analysis and quantitation of cellular lipidomes directly from crude extracts of biological samples. Mass Spectrom Rev 2005. 24:367-412. [DOD] [CrossRef]
- Schwudke D, Liebisch G, Herzog R, Schmitz G, Shevchenko A. Shotgun lipidomics by tandem mass spectrometry under data-dependent acquisition control. Methods Enzymol 2007. 433:175-191. [DOD] [CrossRef]
- Kettunen J, Tukiainen T, Sarin AP, Ortega-Alonso A, Tikkanen E, Lyytikäinen LP, Kangas AJ, Soininen P, Würtz P, Silander K, et al. Genome-wide association study identifies multiple loci influencing human serum metabolite levels. Nat Genet 2012. 44(3):269-276. [DOD] [CrossRef]
- Soininen P, Kangas AJ, Wurtz P, Tukiainen T, Tynkkynen T, Laatikainen R, Järvelin MR, Kähönen M, Lehtimäki T, Viikari J, Raitakari OT, Savolainen MJ, Ala-Korpela M. High-throughput serum NMR metabonomics for cost-effective holistic studies on systemic metabolism. Analyst 2009. 134(9):1781-1785. [DOD] [CrossRef]
- Raffler J, Romisch-Margl W, Petersen AK, Pagel P, Blochl F, Hengstenberg C, Illig T, Meisinger C, Stark K, Wichmann HE, Adamski J, Gieger C, Kastenmuller G, Suhre K. Identification and MS assisted interpretation of genetically influenced NMR signals in human plasma. Genome Med 2013. 5(2):13. [DOD] [CrossRef]
- Castillo S, Mattila I, Miettinen J, Oresic M, Hyotylainen T. Data analysis tool for comprehensive two-dimensional gas chromatography/time-of-flight mass spectrometry. Anal Chem 2011. 83:3058-3067. [DOD] [CrossRef]
- Nygren H, Seppanen-Laakso T, Castillo S, Hyotylainen T, Oresic M. Liquid chromatography-mass spectrometry (LC-MS)-based lipidomics for studies of body fluids and tissues. Methods Mol Biol 2011. 708:247-257. [DOD] [CrossRef]
- Rogers S, Scheltema RA, Girolami M, Breitling R. Probabilistic assignment of formulas to mass peaks in metabolomics experiments. Bioinformatics 2009. 25:512-518. [DOD] [CrossRef]
- Katajamaa M, Oresic M. Data processing for mass spectrometry-based metabolomics. J Chromatogr A 2007. 1158:318-328. [DOD] [CrossRef]
- Pluskal T, Castillo S, Villar-Briones A, Oresic M. MZmine 2: Modular framework for processing, visualizing, and analyzing mass spectrometry-based molecular profile data. BMC Bioinformatics 2010. 11:395. [DOD] [CrossRef]
- Benton HP, Wong DM, Trauger SA, Siuzdak G. XCMS2: processing tandem mass spectrometry data for metabolite identification and structural characterization. Anal Chem 2008. 80:6382-6389. [DOD] [CrossRef]
- Broadhurst D, Kell D. Statistical strategies for avoiding false discoveries in metabolomics and related experiments. Metabolomics 2006. 2:171-196. [DOD] [CrossRef]
- Kankainen M, Gopalacharyulu P, Holm L, Oresic M. MPEA--metabolite pathway enrichment analysis. Bioinformatics 2011. 27:1878-1879. [DOD] [CrossRef]
- Niemela PS, Castillo S, Sysi-Aho M, Oresic M. Bioinformatics and computational methods for lipidomics. J Chromatogr B 2009. 877:2855-2862. [DOD] [CrossRef]
- Pietilainen KH, Rog T, Seppanen-Laakso T, Virtue S, Gopalacharyulu P, Tang J, Rodriguez-Cuenca S, Maciejewski A, Naukkarinen J, Ruskeepää AL, et al. Association of lipidome remodeling in the adipocyte membrane with acquired obesity in humans. Plos Biol 2011. 9(6):e1000623. [DOD] [CrossRef]
- Yetukuri L, Soderlund S, Koivuniemi A, Seppanen-Laakso T, Niemela PS, Hyvönen M, Taskinen MR, Vattulainen I, Jauhiainen M, Oresic M. Composition and lipid spatial distribution of HDL particles in subjects with low and high HDL-cholesterol. J Lipid Res 2010. 51(8):2341-2351. [DOD] [CrossRef]
- Maher B. Personal genomes: the case of the missing heritability. Nature 2008. 456:18-21. [DOD] [CrossRef]
- Meyer-Lindenberg A, Weinberger DR. Intermediate phenotypes and genetic mechanisms of psychiatric disorders. Nat Neurosci 2006. 7:818-827. [DOD] [CrossRef]
- Adamski J, Suhre K. Metabolomics platforms for genome wide association studies-linking the genome to the metabolome. Curr Opin Biotechnol 2013. 24:39-47. [DOD] [CrossRef]
- Gieger C, Geistlinger L, Altmaier E, Hrabe de Angelis M, Kronenberg F, Meitinger T, Mewes HW, Wichmann HE, Weinberger KM, Adamski J, Illig T, Suhre K. Genetics meets metabolomics: a genome-wide association study of metabolite profiles in human serum. PLoS Genet 2008. 4(11):e1000282. [DOD] [CrossRef]
- Illig T, Gieger C, Zhai G, Romisch-Margl W, Wang-Sattler R, Prehn C, Altmaier E, Kastenmüller G, Kato BS, Mewes HW, et al. A genome-wide perspective of genetic variation in human metabolism. Nat Genet 2010. 42(2):137-141. [DOD] [CrossRef]
- Suhre K, Shin SY, Petersen AK, Mohney RP, Meredith D, Wägele B, Altmaier E, Deloukas P, Erdmann J, et al. Human metabolic individuality in biomedical and pharmaceutical research. Nature 2011. 477(7362):54-60. [DOD] [CrossRef]
- Franke A, McGovern DP, Barrett JC, Wang K, Radford-Smith GL, Ahmad T, Lees CW, Balschun T, Lee J, Roberts R, et al. Genome-wide meta-analysis increases to 71 the number of confirmed Crohn's disease susceptibility loci. Nat Genet 2010. 42(12):1118-1125. [DOD] [CrossRef]
- Willer CJ, Sanna S, Jackson AU, Scuteri A, Bonnycastle LL, Clarke R, Heath SC, Timpson NJ, Najjar SS, Stringham HM, et al. Newly identified loci that influence lipid concentrations and risk of coronary artery disease. Nat Genet 2008. 40(2):161-169. [DOD] [CrossRef]
- Bäckhed F, Ley RE, Sonnenburg JL, Peterson DA, Gordon JI. Host-bacterial mutualism in the human intestine. Science 2005. 307:1915-1920. [DOD] [CrossRef]
- Sekirov I, Russell SL, Antunes LC, Finlay BB. Gut microbiota in health and disease. Physiol Rev 2010. 90:859-904. [DOD] [CrossRef]
- Holmes E, Li JV, Marchesi JR, Nicholson JK. Gut microbiota composition and activity in relation to host metabolic phenotype and disease risk. Cell Metab 2012. 16:559-564. [DOD] [CrossRef]
- Velagapudi VR, Hezaveh R, Reigstad CS, Gopalacharyulu P, Yetukuri L, Islam S, Felin J, Perkins R, Boren J, Oresic M, Bäckhed F. The gut microbiota modulates host energy and lipid metabolism in mice. J Lipid Res 2010. 51(5):1101-1112. [DOD] [CrossRef]
- Sayin SI, Wahlström A, Felin J, Jäntti S, Marschall HU, Bamberg K, Angelin B, Hyötyläinen T, Oresic M, Bäckhed F. Gut microbiota regulates bile acid metabolism by reducing the levels of taurobetamuricholic acid, a naturally occurring FXR antagonist. Cell Metab 2013. In press. [DOD]
- Tremaroli V, Backhed F. Functional interactions between the gut microbiota and host metabolism. Nature 2012. 489:242-249. [DOD] [CrossRef]
- Wen L, Ley RE, Volchkov PY, Stranges PB, Avanesyan L, Stonebraker AC, Hu C, Wong FS, Szot GL, Bluestone JA, Gordon JI, Chervonsky AV. Innate immunity and intestinal microbiota in the development of type 1 diabetes. Nature 2008. 455(7216):1109-1113. [DOD] [CrossRef]
- Alam C, Bittoun E, Bhagwat D, Valkonen S, Saari A, Jaakkola U, Eerola E, Huovinen P, Hänninen A. Effects of a germ-free environment on gut immune regulation and diabetes progression in non-obese diabetic (NOD) mice. Diabetologia 2011. 54(6):1398-1406. [DOD] [CrossRef]
- Markle JG, Frank DN, Mortin-Toth S, Robertson CE, Feazel LM, Rolle-Kampczyk U, von Bergen M, McCoy KD, Macpherson AJ, Danska JS. Sex differences in the gut microbiome drive hormone-dependent regulation of autoimmunity. Science 2013. In press. [DOD]
- Sysi-Aho M, Ermolov A, Gopalacharyulu PV, Tripathi A, Seppanen-Laakso T, Maukonen J, Mattila I, Ruohonen ST, Vähätalo L, Yetukuri L, et al. Metabolic regulation in progression to autoimmune diabetes. Plos Comput Biol 2011. 7(10):e1002257. [DOD] [CrossRef]
- Sokol H, Pigneur B, Watterlot L, Lakhdari O, Bermudez-Humaran LG, Gratadoux JJ, Blugeon S, Bridonneau C, Furet JP, Corthier G, et al. Faecalibacterium prausnitzii is an anti-inflammatory commensal bacterium identified by gut microbiota analysis of Crohn disease patients. Proc Natl Acad Sci USA 2008. 105(43):16731-16736. [DOD] [CrossRef]
- Atarashi K, Tanoue T, Shima T, Imaoka A, Kuwahara T, Momose Y, Cheng G, Yamasaki S, Saito T, Ohba Y, et al. Induction of colonic regulatory T cells by indigenous Clostridium species. Science 2011. 331(6015):337-341. [DOD] [CrossRef]
- Brown CT, Davis-Richardson AG, Giongo A, Gano KA, Crabb DB, Mukherjee N, Casella G, Drew JC, Ilonen J, Knip M, et al. Gut microbiome metagenomics analysis suggests a functional model for the development of autoimmunity for type 1 diabetes. PLoS One 2011. 6(10):e25792. [DOD] [CrossRef]
- Giongo A, Gano KA, Crabb DB, Mukherjee N, Novelo LL, Casella G, Drew JC, Ilonen J, Knip M, Hyöty H, et al. Toward defining the autoimmune microbiome for type 1 diabetes. ISME J 2011. 5(1):82-91. [DOD] [CrossRef]
- Nikkila J, Sysi-Aho M, Ermolov A, Seppanen-Laakso T, Simell O, Kaski S, Oresic M. Gender-dependent progression of systemic metabolic states in early childhood. Mol Syst Biol 2008. 4:197. [DOD] [CrossRef]
- Maeba R, Maeda T, Kinoshita M, Takao K, Takenaka H, Kusano J, Yoshimura N, Takeoka Y, Yasuda D, Okazaki T, Teramoto T. Plasmalogens in human serum positively correlate with high-density lipoprotein and decrease with aging. J Atheroscler Thromb 2007. 14(1):12-18. [DOD] [CrossRef]
- Oresic M, Simell S, Sysi-Aho M, Nanto-Salonen K, Seppanen-Laakso T, Parikka V, Katajamaa M, Hekkala A, Mattila I, Keskinen P, et al. Dysregulation of lipid and amino acid metabolism precedes islet autoimmunity in children who later progress to type 1 diabetes. J Exp Med 2008. 205(13):2975-2984. [DOD] [CrossRef]
- Pflueger M, Seppanen-Laakso T, Suortti T, Hyotylainen T, Achenbach P, Bonifacio E, Oresic M, Ziegler AG. Age- and islet autoimmunity-associated differences in amino Acid and lipid metabolites in children at risk for type 1 diabetes. Diabetes 2011. 60(11):2740-2747. [DOD] [CrossRef]
- Holmes E, Loo RL, Stamler J, Bictash M, Yap IK, Chan Q, Ebbels T, De Iorio M, Brown IJ, Veselkov KA, Daviglus ML, Kesteloot H, Ueshima H, Zhao L, Nicholson JK, Elliott P. Human metabolic phenotype diversity and its association with diet and blood pressure. Nature 2008. 453(7193):396-400. [DOD] [CrossRef]
- Bougneres P, Valleron AJ. Causes of early-onset type 1 diabetes: toward data-driven environmental approaches. J Exp Med 2008. 205:2953-2957. [DOD] [CrossRef]
- Sreekumar A, Poisson LM, Rajendiran TM, Khan AP, Cao Q, Yu J, Laxman B, Mehra R, Lonigro RJ, Li Y, et al. Metabolomic profiles delineate potential role for sarcosine in prostate cancer progression. Nature 2009. 457(7231):910-914. [DOD] [CrossRef]
- Wang TJ, Larson MG, Vasan RS, Cheng S, Rhee EP, McCabe E, Lewis GD, Fox CS, Jacques PF, Fernandez C, et al. Metabolite profiles and the risk of developing diabetes. Nat Med 2011. 17(4):448-453. [DOD] [CrossRef]
- Rhee EP, Cheng S, Larson MG, Walford GA, Lewis GD, McCabe E, Yang E, Farrell L, Fox CS, O'Donnell CJ, Carr SA, Vasan RS, Florez JC, Clish CB, Wang TJ, Gerszten RE. Lipid profiling identifies a triacylglycerol signature of insulin resistance and improves diabetes prediction in humans. J Clin Invest 2011. 121(4):1402-1411. [DOD] [CrossRef]
- Gall WE, Beebe K, Lawton KA, Adam KP, Mitchell MW, Nakhle PJ, Ryals JA, Milburn MV, Nannipieri M, Camastra S, et al. Alpha-hydroxybutyrate is an early biomarker of insulin resistance and glucose intolerance in a nondiabetic population. Plos One 2010. 5(5):e10883. [DOD] [CrossRef]
- Oresic M, Hyötyläinen T, Herukka SK, Sysi-Aho M, Mattila I, Seppänan-Laakso T, Julkunen V, Gopalacharyulu PV, Hallikainen M, Koikkalainen J, Kivipelto M, Helisalmi S, Lötjönen J, Soininen H. Metabolome in progression to Alzheimer's disease. Transl Psychiatry 2011. 1:e57. [DOD] [CrossRef]
- Frezza C, Zheng L, Folger O, Rajagopalan KN, MacKenzie ED, Jerby L, Micaroni M, Chaneton B, Adam J, Hedley A, et al. Haem oxygenase is synthetically lethal with the tumour suppressor fumarate hydratase. Nature 2011. 477(7363):225-228. [DOD] [CrossRef]
- McNamara LE, Sjostrom T, Meek RM, Oreffo RO, Su B, Dalby MJ, Burgess KE. Metabolomics: a valuable tool for stem cell monitoring in regenerative medicine. J R Soc Interface 2012. 9(73):1713-1724. [DOD] [CrossRef]
- O'Brien PJ, Lee M, Spilker ME, Zhang CC, Yan Z, et al. Monitoring metabolic responses to chemotherapy in single cells and tumors using nanostructure-initiator mass spectrometry (NIMS) imaging. Cancer Metab 2013. 1:e4. [DOD] [CrossRef]
- Bain JR, Stevens RD, Wenner BR, Ilkayeva O, Muoio DM, Newgard CB. Metabolomics applied to diabetes research: moving from information to knowledge. Diabetes 2009. 58(11):2429-2443. [DOD] [CrossRef]
- Zeisel SH. Choline: Critical role during fetal development and dietary requirements in adults. Annu Rev Nutr 2006. 26:229-250. [DOD] [CrossRef]
- Jaenisch R, Bird A. Epigenetic regulation of gene expression: how the genome integrates intrinsic and environmental signals. Nat Genet 2003. 33(Suppl):245-254. [DOD] [CrossRef]
- Madsen R, Banday VS, Moritz T, Trygg J, Lejon K. Altered metabolic signature in pre-diabetic NOD mice. Plos One 2012. 7:e35445. [DOD] [CrossRef]
- Makinen VP, Soininen P, Forsblom C, Parkkonen M, Ingman P, Kaski K, Groop PH, FinnDiane Study Group, Ala-Korpela M. 1H NMR metabonomics approach to the disease continuum of diabetic complications and premature death. Mol Syst Biol 2008. 4:167. [DOD] [CrossRef]
- Makinen VP, Tynkkynen T, Soininen P, Forsblom C, Peltola T, Kangas AJ, Groop PH, Ala-Korpela M. Sphingomyelin is associated with kidney disease in type 1 diabetes (The FinnDiane Study). Metabolomics 2012. 8(3):369-375. [DOD] [CrossRef]
- van der Kloet FM, Tempels FW, Ismail N, van der Heijden R, Kasper PT, Rojas-Cherto M, van Doorn R, Spijksma G, Koek M, van der Greef J, Mäkinen VP, Forsblom C, Holthöfer H, Groop PH, Reijmers TH, Hankemeier T. Discovery of early-stage biomarkers for diabetic kidney disease using ms-based metabolomics (FinnDiane study). Metabolomics 2012. 8(1):109-119. [DOD] [CrossRef]
- Makinen VP, Tynkkynen T, Soininen P, Peltola T, Kangas AJ, Forsblom C, Thorn LM, Kaski K, Laatikainen R, Ala-Korpela M, Groop PH. Metabolic diversity of progressive kidney disease in 325 patients with type 1 diabetes (the FinnDiane Study). J Proteome Res 2012. 11(3):1782-1790. [DOD] [CrossRef]
- Thomas MC, Soderlund J, Lehto M, Makinen VP, Moran JL, Cooper ME, Forsblom C, Groop PH, FinnDiane Study Group. Soluble receptor for AGE (RAGE) is a novel independent predictor of all-cause and cardiovascular mortality in type 1 diabetes. Diabetologia 2011. 54(10):2669-2677. [DOD] [CrossRef]
- Hauben E, Roncarolo MG, Nevo U, Schwartz M. Beneficial autoimmunity in type 1 diabetes mellitus. Trends Immunol 2005. 26:248-253. [DOD] [CrossRef]
- Marinkovic T, Sysi-Aho M, Oresic M. Integrated model of metabolism and autoimmune response in beta-cell death and progression to type 1 diabetes. Plos One 2012. 7:e51909. [DOD] [CrossRef]
- Korte SM, Koolhaas JM, Wingfield JC, McEwen BS. The Darwinian concept of stress: benefits of allostasis and costs of allostatic load and the trade-offs in health and disease. Neurosci Biobehav Rev 2005. 29:3-38. [DOD] [CrossRef]
- Knip M, Veijola R, Virtanen SM, Hyöty H, Vaarala O, Akerblom HK. Environmental triggers and determinants of type 1 diabetes. Diabetes 2005. 54(Suppl 2):S125-S136. [DOD] [CrossRef]
- Freiesleben De Blasio B, Bak P, Pociot F, Karlsen AE, Nerup J. Onset of type 1 diabetes: a dynamical instability. Diabetes 1999. 48:1677-1685. [DOD] [CrossRef]
This article has been cited by other articles:
|
Metabolomics reveals new metabolic perturbations in children with type 1 diabetes
Galderisi A, Pirillo P, Moret V, Stocchero M, Gucciardi A, Perilongo G, Moretti C, Monciotti C, Giordano G, Baraldi E
Pediatr Diabetes 2017. In press
|
|
|
Tolerogenic Dendritic Cells from Poorly Compensated Type 1 Diabetes Patients Have Decreased Ability To Induce Stable Antigen-Specific T Cell Hyporesponsiveness and Generation of Suppressive Regulatory T Cells
Danova K, Grohova A, Strnadova P, Funda DP, Sumnik Z, Lebl J, Cinek O, Pruhova S, Kolouskova S, Obermannova B, Petruzelkova L, Sediva A, Fundova P, Buschard K, Spisek R, Palova-Jelinkova L
J Immunol 2017. 198(2):729-740
|
|
|
Mitochondrial aldehyde dehydrogenase 2 deficiency aggravates energy metabolism disturbance and diastolic dysfunction in diabetic mice
Wang C, Fan F, Cao Q, Shen C, Zhu H, Wang P, Zhao X, Sun X, Dong Z, Ma X, Liu X, Han S, Wu C, Zou Y, Hu K, Ge J, Sun A
J Mol Med (Berl) 2016. 94(11):1229-1240
|
|
|
Metabolomics applied to the pancreatic islet
Gooding JR, Jensen MV, Newgard CB
Arch Biochem Biophys 2016. 589:120-130
|
|
|
Modeling strategies to study metabolic pathways in progression to type 1 diabetes - Challenges and opportunities
Marinkovic T, Oresic M
Arch Biochem Biophys 2016. 589:131-137
|
|
|
Metabolomics in childhood diabetes
Frohnert BI, Rewers MJ
Pediatr Diabetes 2016. 17(1):3-14
|
|
|
Staging presymptomatic type 1 diabetes: a scientific statement of JDRF, the Endocrine Society, and the American Diabetes Association
Insel RA, Dunne JL, Atkinson MA, Chiang JL, Dabelea D, Gottlieb PA, Greenbaum CJ, Herold KC, Krischer JP, Lernmark A, Ratner RE, Rewers MJ, Schatz DA, Skyler JS, Sosenko JM, Ziegler AG
Diabetes Care 2015. 38(10):1964-1974
|
|
|
Heterogeneity in diabetes-associated autoantibodies and susceptibility to Type 1 diabetes: lessons for disease prevention
Ilonen J, Knip M, Vaarala O
Exp Rev Endocrinol Metab 2015. 10(1):25-34
|
|
|
Systems biology strategies to study lipidomes in health and disease
Hyötyläinen T, Oresic M
Prog Lipid Res 2014. 55:43-60
|
|
|